base on Official code for "FeatUp: A Model-Agnostic Frameworkfor Features at Any Resolution" ICLR 2024 # FeatUp: A Model-Agnostic Framework for Features at Any Resolution
### ICLR 2024
[](https://aka.ms/featup) [](https://arxiv.org/abs/2403.10516) [](https://colab.research.google.com/github/mhamilton723/FeatUp/blob/main/example_usage.ipynb)
[](https://huggingface.co/spaces/mhamilton723/FeatUp)
[](https://huggingface.co/papers/2403.10516)
[](https://paperswithcode.com/sota/feature-upsampling-on-imagenet?p=featup-a-model-agnostic-framework-for)
[Stephanie Fu*](https://stephanie-fu.github.io/),
[Mark Hamilton*](https://mhamilton.net/),
[Laura Brandt](https://people.csail.mit.edu/lebrandt/),
[Axel Feldman](https://feldmann.nyc/),
[Zhoutong Zhang](https://ztzhang.info/),
[William T. Freeman](https://billf.mit.edu/about/bio)
*Equal Contribution.
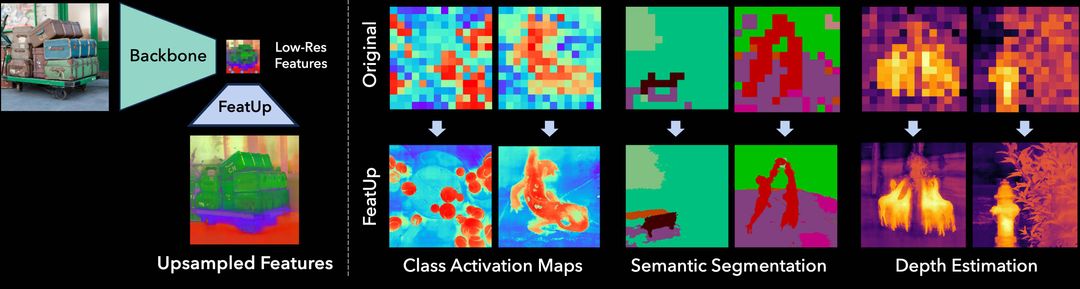
*TL;DR*:FeatUp improves the spatial resolution of any model's features by 16-32x without changing their semantics.
https://github.com/mhamilton723/FeatUp/assets/6456637/8fb5aa7f-4514-4a97-aebf-76065163cdfd
## Contents
<!--ts-->
* [Install](#install)
* [Using Pretrained Upsamplers](#using-pretrained-upsamplers)
* [Fitting an Implicit Upsampler](#fitting-an-implicit-upsampler-to-an-image)
* [Coming Soon](coming-soon)
* [Citation](#citation)
* [Contact](#contact)
<!--te-->
## Install
### Pip
For those just looking to quickly use the FeatUp APIs install via:
```shell script
pip install git+https://github.com/mhamilton723/FeatUp
```
### Local Development
To install FeatUp for local development and to get access to the sample images install using the following:
```shell script
git clone https://github.com/mhamilton723/FeatUp.git
cd FeatUp
pip install -e .
```
## Using Pretrained Upsamplers
To see examples of pretrained model usage please see our [Collab notebook](https://colab.research.google.com/github/mhamilton723/FeatUp/blob/main/example_usage.ipynb). We currently supply the following pretrained versions of FeatUp's JBU upsampler:
| Model Name | Checkpoint | Checkpoint (No LayerNorm) | Torch Hub Repository | Torch Hub Name |
|------------|----------------------------------------------------------------------------------------------------------------------------------|--------------------------------------------------------------------------------------------------------------------------------------------|----------------------|----------------|
| DINO | [Download](https://marhamilresearch4.blob.core.windows.net/feature-upsampling-public/pretrained/dino16_jbu_stack_cocostuff.ckpt) | [Download](https://marhamilresearch4.blob.core.windows.net/feature-upsampling-public/pretrained/no_norm/dino16_jbu_stack_cocostuff.ckpt) | mhamilton723/FeatUp | dino16 |
| DINO v2 | [Download](https://marhamilresearch4.blob.core.windows.net/feature-upsampling-public/pretrained/dinov2_jbu_stack_cocostuff.ckpt) | [Download](https://marhamilresearch4.blob.core.windows.net/feature-upsampling-public/pretrained/no_norm/dinov2_jbu_stack_cocostuff.ckpt) | mhamilton723/FeatUp | dinov2 |
| CLIP | [Download](https://marhamilresearch4.blob.core.windows.net/feature-upsampling-public/pretrained/clip_jbu_stack_cocostuff.ckpt) | [Download](https://marhamilresearch4.blob.core.windows.net/feature-upsampling-public/pretrained/no_norm/clip_jbu_stack_cocostuff.ckpt) | mhamilton723/FeatUp | clip |
| MaskCLIP | n/a | [Download](https://marhamilresearch4.blob.core.windows.net/feature-upsampling-public/pretrained/no_norm/maskclip_jbu_stack_cocostuff.ckpt) | mhamilton723/FeatUp | maskclip |
| ViT | [Download](https://marhamilresearch4.blob.core.windows.net/feature-upsampling-public/pretrained/vit_jbu_stack_cocostuff.ckpt) | [Download](https://marhamilresearch4.blob.core.windows.net/feature-upsampling-public/pretrained/no_norm/vit_jbu_stack_cocostuff.ckpt) | mhamilton723/FeatUp | vit |
| ResNet50 | [Download](https://marhamilresearch4.blob.core.windows.net/feature-upsampling-public/pretrained/resnet50_jbu_stack_cocostuff.ckpt) | [Download](https://marhamilresearch4.blob.core.windows.net/feature-upsampling-public/pretrained/no_norm/resnet50_jbu_stack_cocostuff.ckpt) | mhamilton723/FeatUp | resnet50 |
For example, to load the FeatUp JBU upsampler for the DINO backbone without an additional LayerNorm on the spatial features:
```python
upsampler = torch.hub.load("mhamilton723/FeatUp", 'dino16', use_norm=False)
```
To load upsamplers trained on backbones with additional LayerNorm operations which makes training and transfer learning a bit more stable:
```python
upsampler = torch.hub.load("mhamilton723/FeatUp", 'dino16')
```
## Fitting an Implicit Upsampler to an Image
To train an implicit upsampler for a given image and backbone first clone the repository and install it for
[local development](#local-development). Then run
```python
cd featup
python train_implicit_upsampler.py
```
Parameters for this training operation can be found in the [implicit_upsampler config file](featup/configs/implicit_upsampler.yaml).
## Local Gradio Demo
To run our [HuggingFace Spaces hosted FeatUp demo](https://huggingface.co/spaces/mhamilton723/FeatUp) locally first install FeatUp for local development. Then run:
```shell
python gradio_app.py
```
Wait a few seconds for the demo to spin up, then navigate to [http://localhost:7860/](http://localhost:7860/) to view the demo.
## Coming Soon:
- Training your own FeatUp joint bilateral upsampler
- Simple API for Implicit FeatUp training
## Citation
```
@inproceedings{
fu2024featup,
title={FeatUp: A Model-Agnostic Framework for Features at Any Resolution},
author={Stephanie Fu and Mark Hamilton and Laura E. Brandt and Axel Feldmann and Zhoutong Zhang and William T. Freeman},
booktitle={The Twelfth International Conference on Learning Representations},
year={2024},
url={https://openreview.net/forum?id=GkJiNn2QDF}
}
```
## Contact
For feedback, questions, or press inquiries please contact [Stephanie Fu](mailto:
[email protected]) and [Mark Hamilton](mailto:
[email protected])
", Assign "at most 3 tags" to the expected json: {"id":"8737","tags":[]} "only from the tags list I provide: [{"id":77,"name":"3d"},{"id":89,"name":"agent"},{"id":17,"name":"ai"},{"id":54,"name":"algorithm"},{"id":24,"name":"api"},{"id":44,"name":"authentication"},{"id":3,"name":"aws"},{"id":27,"name":"backend"},{"id":60,"name":"benchmark"},{"id":72,"name":"best-practices"},{"id":39,"name":"bitcoin"},{"id":37,"name":"blockchain"},{"id":1,"name":"blog"},{"id":45,"name":"bundler"},{"id":58,"name":"cache"},{"id":21,"name":"chat"},{"id":49,"name":"cicd"},{"id":4,"name":"cli"},{"id":64,"name":"cloud-native"},{"id":48,"name":"cms"},{"id":61,"name":"compiler"},{"id":68,"name":"containerization"},{"id":92,"name":"crm"},{"id":34,"name":"data"},{"id":47,"name":"database"},{"id":8,"name":"declarative-gui "},{"id":9,"name":"deploy-tool"},{"id":53,"name":"desktop-app"},{"id":6,"name":"dev-exp-lib"},{"id":59,"name":"dev-tool"},{"id":13,"name":"ecommerce"},{"id":26,"name":"editor"},{"id":66,"name":"emulator"},{"id":62,"name":"filesystem"},{"id":80,"name":"finance"},{"id":15,"name":"firmware"},{"id":73,"name":"for-fun"},{"id":2,"name":"framework"},{"id":11,"name":"frontend"},{"id":22,"name":"game"},{"id":81,"name":"game-engine "},{"id":23,"name":"graphql"},{"id":84,"name":"gui"},{"id":91,"name":"http"},{"id":5,"name":"http-client"},{"id":51,"name":"iac"},{"id":30,"name":"ide"},{"id":78,"name":"iot"},{"id":40,"name":"json"},{"id":83,"name":"julian"},{"id":38,"name":"k8s"},{"id":31,"name":"language"},{"id":10,"name":"learning-resource"},{"id":33,"name":"lib"},{"id":41,"name":"linter"},{"id":28,"name":"lms"},{"id":16,"name":"logging"},{"id":76,"name":"low-code"},{"id":90,"name":"message-queue"},{"id":42,"name":"mobile-app"},{"id":18,"name":"monitoring"},{"id":36,"name":"networking"},{"id":7,"name":"node-version"},{"id":55,"name":"nosql"},{"id":57,"name":"observability"},{"id":46,"name":"orm"},{"id":52,"name":"os"},{"id":14,"name":"parser"},{"id":74,"name":"react"},{"id":82,"name":"real-time"},{"id":56,"name":"robot"},{"id":65,"name":"runtime"},{"id":32,"name":"sdk"},{"id":71,"name":"search"},{"id":63,"name":"secrets"},{"id":25,"name":"security"},{"id":85,"name":"server"},{"id":86,"name":"serverless"},{"id":70,"name":"storage"},{"id":75,"name":"system-design"},{"id":79,"name":"terminal"},{"id":29,"name":"testing"},{"id":12,"name":"ui"},{"id":50,"name":"ux"},{"id":88,"name":"video"},{"id":20,"name":"web-app"},{"id":35,"name":"web-server"},{"id":43,"name":"webassembly"},{"id":69,"name":"workflow"},{"id":87,"name":"yaml"}]" returns me the "expected json"